Artificial intelligence could lead to an Orwellian future if laws to protect the public aren't enacted soon, according to Microsoft President Brad Smith.
Smith made the comments to the BBC news program "Panorama" on May 26, during an episode focused on the potential dangers of artificial intelligence (AI) and the race between the United States and China to develop the technology. The warning comes about a month after the European Union released draft regulations attempting to set limits on how AI can be used. There are few similar efforts in the United States, where legislation has largely focused on limiting regulation and promoting AI for national security purposes.
"I'm constantly reminded of George Orwell's lessons in his book '1984,'" Smith said. "The fundamental story was about a government that could see everything that everyone did and hear everything that everyone said all the time. Well, that didn't come to pass in 1984, but if we're not careful, that could come to pass in 2024."
A tool with a dark side
Artificial intelligence is an ill-defined term, but it generally refers to machines that can learn or solve problems automatically, without being directed by a human operator. Many AI programs today rely on machine learning, a suite of computational methods used to recognize patterns in large amounts of data and then apply those lessons to the next round of data, theoretically becoming more and more accurate with each pass.
This is an extremely powerful approach that has been applied to everything from basic mathematical theory to simulations of the early universe, but it can be dangerous when applied to social data, experts argue. Data on humans comes preinstalled with human biases. For example, a recent study in the journal JAMA Psychiatry found that algorithms meant to predict suicide risk performed far worse on Black and American Indian/Alaskan Native individuals than on white individuals, partially because there were fewer patients of color in the medical system and partially because patients of color were less likely to get treatment and appropriate diagnoses in the first place, meaning the original data was skewed to underestimate their risk.
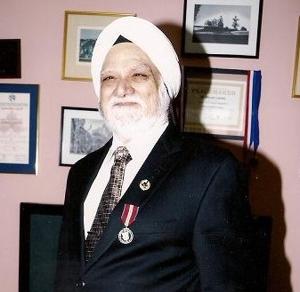
Write a comment ...